advice
Effective Data Teams: 6 Key Challenges for Businesses
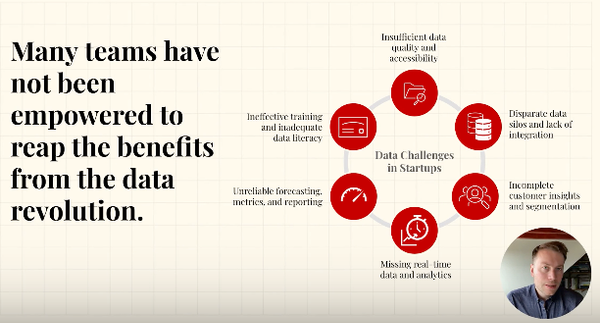
Invested a lot in data over the years? But struggling to see results? You’re not alone. Building data teams is hard, and getting them to be a profit centre (rather than a cost) is even harder.
So! Let’s make this more concrete and talk about six key problems most data teams face — and what you can do about them.
1. Data Quality Matters
One of the most important aspects of effective data use is ensuring high data quality. Poor data quality can lead to incorrect insights, misguided strategies, and wasted resources. Clean and reliable data is essential for understanding customer behaviour, optimising marketing campaigns, and managing inventory efficiently. Explore tools like Avo and Trackingplan to get better at this — and please make sure you think about data quality before gathering and ingesting data!
Example: An e-commerce company discovers that its marketing data shows inconsistent customer names and email addresses due to typographical errors and duplicated entries. As a result, personalised marketing campaigns are less effective, and customer engagement suffers. To address this, the company invests in data governance tools and processes, ensuring that all data entering their system is accurate and standardised.
By investing upfront in data quality, the company can avoid costly errors and build a solid foundation for all subsequent data initiatives.
2. Align Definitions Across Teams
Standardising key metrics and definitions across departments is crucial to ensure everyone in the organisation is aligned and working towards the same goals. In many cases, different teams might have different definitions for common metrics, leading to confusion and inefficiency.
Example: The marketing team at the e-commerce company defines a “customer” as anyone who has subscribed to their newsletter, while the sales team considers a “customer” to be someone who has made a purchase. This discrepancy leads to misaligned strategies and reporting. By holding cross-departmental workshops, the company agrees on a unified definition of key metrics, ensuring that everyone is on the same page.
This alignment helps in creating coherent strategies and improving communication across teams, ultimately leading to better decision-making.
3. Invest in Proper Data Infrastructure
A robust data infrastructure is the backbone of effective data management. It enables the seamless integration, storage, and retrieval of data from various sources. For an e-commerce company, this means having a system that can handle data from marketing platforms, customer interactions, and supply chain systems.
Example: The e-commerce company uses multiple marketing tools, a CRM for customer data, and an ERP system for supply chain management. Initially, these systems are siloed, making it difficult to get a comprehensive view of the business. By investing in a centralised data warehouse, the company can integrate data from all these sources, providing a unified view of operations.
This centralised approach not only simplifies data management but also enhances the ability to perform advanced analytics and generate valuable insights.
4. Technical Challenges
Technical issues often hinder the effective use of data. These challenges can range from integrating diverse data sources to managing large volumes of data and ensuring data security.
Example: The e-commerce company struggles with integrating data from its social media marketing campaigns with customer purchase data. The marketing team uses different platforms with varying data formats and structures. By implementing an ETL (Extract, Transform, Load) process, the company can automatically extract data from various sources, transform it into a consistent format, and load it into the data warehouse.
Addressing these technical challenges not only improves data integration but also ensures that the data is ready for analysis and decision-making.
5. Cost vs. Profit Centre
Traditionally, data teams are seen as cost centres within organisations. However, by using analytics to drive revenue and efficiency, companies can transform their data teams into profit centres.
Example: The e-commerce company’s data team initially focuses on generating reports and maintaining data infrastructure, which are seen as cost activities. By shifting their focus to predictive analytics, they can provide insights into customer behaviour, optimise marketing spend, and improve inventory management. For instance, predicting which products are likely to be popular during the holiday season can help in better inventory planning, reducing stockouts and overstock situations.
This proactive approach demonstrates the value of the data team, showing how their work directly contributes to the company’s bottom line.
6. Experience and Expertise
Drawing from the experiences of industry experts can significantly enhance a company’s data strategy. Learning from the successes and failures of others helps in implementing best practices and avoiding common pitfalls.
Example: The e-commerce company partners with a consulting firm that has extensive experience in data transformation. The consultants share their insights from working with other e-commerce companies, providing practical advice on data integration, quality management, and advanced analytics. They help the company implement a machine learning model to personalise product recommendations, resulting in increased sales and improved customer satisfaction.
By leveraging external expertise, the company accelerates its data transformation journey, adopting proven strategies and avoiding costly mistakes.
Data As A North Star
Getting to a great data team is challenging but immensely rewarding. By focusing on data quality, aligning definitions across teams, investing in proper data infrastructure, addressing technical challenges, shifting the perspective of data teams from cost centres to profit centres, and leveraging external expertise, you will have solved most of the potential pitfalls along the way.
For the ecommerce company we illustrated this with, these steps are crucial in integrating marketing, customer, and supply chain data, ultimately leading to better decision-making, improved customer experiences, and increased profitability.